New AI algorithm scans patient records to find undiagnosed AHP
Goal is to use artificial intelligence tool to diagnose rare diseases sooner
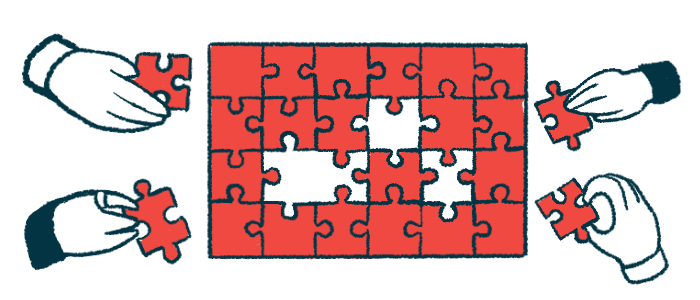
A new artificial intelligence (AI) algorithm may help reduce the time it takes to diagnose acute hepatic porphyria (AHP) and other rare diseases.
That’s according to a study reporting that models using the AI algorithm “could identify 71% of [AHP] cases earlier than their diagnosis date, saving 1.2 years.”
Despite these promising results, more extensive testing is needed to ensure the algorithm is accurate and reliable before it can be widely used, the researchers noted.
The algorithm is the brainchild of University of California Los Angeles (UCLA) David Geffen School of Medicine graduate Katharina “Kat” Schmolly, MD, who also launched an AI-powered platform called ZebraMD to help flag patients who, based on their electronic health records, may have rare diseases.
“The diagnostic delay is roughly 10 to 15 years for these diseases because physicians don’t see them very often,” Schmolly said in a UCLA Health news story. “While waiting for diagnosis, the disease can progress and cause irreversible damage. So, our goal is to diagnose patients earlier and manage their disease appropriately.”
The study, “Reducing diagnostic delays in acute hepatic porphyria using health records data and machine learning,” was published in the Journal of the American Medical Informatics Association. It was funded in part by Alnylam Pharmaceuticals, the company that markets Givlaari (givosiran), an approved treatment for adults with AHP.
Then-UCLA med student worked with UCSF professor on AI algorithm
Porphyria occurs when the body is missing one of the enzymes that take part in the chemical steps used to make a molecule called heme, a component of hemoglobin, which is involved in oxygen transport. The shortage of one of these enzymes causes heme building blocks to go unused and build up to toxic levels in the body’s organs. In AHP, they build up in the liver.
Symptoms, which include abdominal pain, constipation, and anxiety, appear suddenly and can last hours to days. In women, complaints often coincide with the menstrual cycle, which can make a diagnosis difficult.
“Women unfortunately get dismissed when they go to the emergency department over and over again for these complaints,” Schmolly said. “Because it looks like it’s menstrual pain, but actually, it could be a true liver disease.”
Electronic health records and machine learning, a branch of AI that learns patterns from data to make predictions, offer opportunities for a more timely diagnosis of rare diseases, such as AHP. But it can be difficult to train an algorithm given the limited number of patients with these conditions.
Against this backdrop, Schmolly worked in collaboration with Vivek Rudrapatna, MD, PhD, an assistant professor at the University of California, San Francisco (UCSF). The duo developed an algorithm they called Project Zebra that could flag patients suspected of having AHP. The zebra is a mascot for people with rare diseases, as noted by the National Organization for Rare Diseases.
Data to feed the algorithm were pooled from UCSF and UCLA de-identified patient records.
“Given that it’s a rare disease, we knew up front that it was going to be difficult or impossible to run this as a single center endeavor. So we looked to our peers within the UC health network,” Rudrapatna said.
Model could possibly ‘reduce diagnostic delays in AHP and other rare diseases’
The data were divided into two groups to develop a machine learning model that could predict who would be referred for AHP testing among those who presented with abdominal pain, and who would test positive for the disease among those referred.
The referral group included 747 patients who had been recommended for testing and 99,849 patients who had not. The diagnosis group included 72 patients, ages 6-75 and mostly female, with a confirmed diagnosis of AHP and 347 patients who tested negative.
The model predicted which patients would be referred for testing with an accuracy of up to more than 90%. Moreover, it was estimated that it could flag 71% of patients an average of 1.2 years earlier than their diagnosis. Machine learning may therefore “reduce diagnostic delays in AHP and other rare diseases,” the researchers wrote.
To build the model, Schmolly and Rudrapatna drew on expert knowledge from Bruce Wang, MD, director of the UCSF Porphyria Center, who shared information on symptoms. They also provided access to a rare diseases database from the National Institutes of Health.
The more patients we can diagnose, the more we can monitor over time. … That means we can learn what works and what doesn’t work. We can create precision medicine approaches for these rare diseases.
Project Zebra plans to soon launch a web app developed by Kristen Cardon, an English doctoral candidate at UCLA and a software engineering intern at ZebraMD. If all goes as planned, ZebraMD will begin testing its algorithms in the real world later this year by embedding into electronic health records systems.
“Someone might come here looking for information about their own diagnosis,” Cardon said. “At a time that could be scary and alarming, our app is just making information accessible, easy and functional.”
Schmolly, who will continue to lead her team at ZebraMD during her internal medicine residency at Dartmouth Health in New Hampshire, hopes “that at some point this is a standard feature of any electronic health records system.”
“The more patients we can diagnose, the more we can monitor over time,” Schmolly said. “That means we can learn what works and what doesn’t work. We can create precision medicine approaches for these rare diseases. Many of them don’t have any treatments yet.”